BSU Virtual Seminar: ‘Parameterizing Causal Marginal Models’
Duration: 52 mins 4 secs
Share this media item:
Embed this media item:
Embed this media item:
About this item
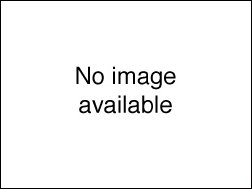
Description: |
Speaker: Prof Robin Evans, University of Oxford
Title: ‘Parameterizing Causal Marginal Models’ |
---|
Created: | 2020-06-16 15:35 |
---|---|
Collection: | BSU Virtual Seminars 2020 |
Publisher: | University of Cambridge |
Copyright: | A.S. Quenault |
Language: | eng (English) |
Distribution: |
World
![]() |
Keywords: | Statistics; Biology; Public Health; Mathematics; |
Explicit content: | No |
Aspect Ratio: | 16:9 |
Screencast: | No |
Bumper: | UCS Default |
Trailer: | UCS Default |
Abstract: | Many statistical problems in causal inference involve a probability distribution other than the one from which data are actually observed; as an additional complication, the quantity of interest is often a marginal quantity of this other probability distribution. This creates many practical complications for statistical inference, even where the problem is non-parametrically identified.
Naive attempts to specify a model parametrically can lead to unwanted consequences such as incompatible parametric assumptions or the so-called ‘g-null paradox’. As a consequence it is difficult to perform likelihood-based inference, or even to simulate from the model in a general way. We argue that this occurs because insufficient thought is given to which parts of the model are truly of interest, what nuisance parameters remain to be specified given the parts of interest, and what other aspects of the distribution are redundant and can be derived from the rest. We provide a recipe for constructing a smooth, non-redundant parameterization using (identifiable) causal quantities of interest. We adapt some existing marginal parameterizations to causal models for both continuous and discrete data, allowing us to parameterize a wide range of causal models including marginal structural models (MSMs), Cox MSMs and structural nested models. This makes it easy to simulate from and fit models; to introduce possibly high-dimensional individual-level covariates; and to include additional assumptions such as stationarity or symmetry. Our approach is likelihood-based, and therefore also amenable to a fully Bayesian approach. We also provide an R package that implements these routines. |
---|